Hate Speech Detection And Sentiment Analysis Using Machine Learning And Deep Learning

4.6 out of 5
Language | : | English |
File size | : | 5502 KB |
Text-to-Speech | : | Enabled |
Enhanced typesetting | : | Enabled |
Print length | : | 221 pages |
Lending | : | Enabled |
Screen Reader | : | Supported |
Unveiling the Truths
In an era defined by ubiquitous digital communication, the internet has become a fertile ground for the dissemination of hateful and offensive content. Hate speech, characterized by its intent to incite hatred or violence against individuals or groups based on their protected characteristics, poses significant threats to social cohesion and individual well-being.
Sentiment analysis, the automated process of identifying and understanding the emotional tone of text, offers a powerful tool for analyzing public sentiment and gauging the overall mood of online conversations. By harnessing the capabilities of machine learning and deep learning, researchers and practitioners are developing innovative solutions to detect hate speech and perform sentiment analysis with unprecedented accuracy and efficiency.
Challenges in Hate Speech Detection and Sentiment Analysis
Despite the significant advancements in natural language processing (NLP),hate speech detection and sentiment analysis remain challenging tasks due to several factors:
- Contextual Ambiguity: Hate speech often relies on subtle cues and context-dependent meanings, making it difficult for machines to accurately interpret the intended message.
- Sarcasm and Irony: Hateful content can be expressed through sarcasm or irony, which can confuse traditional NLP models.
- Implicit Bias: Machine learning models can inherit biases from the training data, leading to unfair or inaccurate results.
- Evolving Language: Hate speech and online communication patterns are constantly evolving, requiring models to adapt to new forms of expression.
Machine Learning and Deep Learning for Hate Speech Detection
Machine learning algorithms, trained on large datasets of labeled speech, have proven effective in detecting hate speech. Supervised learning techniques, such as support vector machines (SVMs) and logistic regression, have been widely used for this task.
Deep learning models, particularly convolutional neural networks (CNNs) and recurrent neural networks (RNNs),have shown even greater promise in hate speech detection. These models can capture complex patterns and relationships in text, leading to improved accuracy and robustness.
Machine learning approaches for hate speech detection typically involve the following steps:
- Data Collection and Labeling: A large and diverse dataset of labeled hate speech and non-hate speech text is collected.
- Feature Extraction: Textual features, such as bag-of-words, n-grams, and word embeddings, are extracted from the text.
- Model Training: A machine learning model is trained on the labeled dataset to learn the patterns associated with hate speech.
- Model Evaluation: The trained model is evaluated on a held-out test dataset to assess its performance.
Deep Learning for Sentiment Analysis
Deep learning models have revolutionized sentiment analysis by enabling the automatic extraction of semantic features from text. Convolutional neural networks (CNNs) and recurrent neural networks (RNNs) have been particularly successful in this domain.
Deep learning approaches for sentiment analysis typically involve the following steps:
- Data Collection and Labeling: A dataset of labeled text with known sentiment polarity (e.g., positive, negative, neutral) is collected.
- Text Preprocessing: The text is preprocessed to remove noise and extract relevant features.
- Model Training: A deep learning model is trained on the labeled dataset to learn the patterns associated with different sentiment polarities.
- Model Evaluation: The trained model is evaluated on a held-out test dataset to assess its performance.
Real-World Applications of Hate Speech Detection and Sentiment Analysis
The advancements in hate speech detection and sentiment analysis have far-reaching implications for various real-world applications:
- Social Media Monitoring: Social media platforms can leverage these technologies to identify and remove hateful content, promoting a safer and more inclusive online environment.
- Online Safety: Hate speech detection and sentiment analysis can be used to protect individuals from online harassment, cyberbullying, and hate crimes.
- Law Enforcement: Law enforcement agencies can use these technologies to investigate hate crimes and identify potential threats.
- Public Policy: Governments and policymakers can use these technologies to understand the prevalence of hate speech and develop effective countermeasures.
The combination of machine learning and deep learning has revolutionized hate speech detection and sentiment analysis, enabling the development of highly accurate and robust models. These technologies have the potential to transform the way we interact online, making the internet a safer and more inclusive space for all.
While these technologies offer immense promise, it is crucial to address the ethical and societal implications of their use. Bias mitigation, fairness, and transparency should be at the forefront of research and development efforts to ensure that these technologies are used responsibly and equitably.
4.6 out of 5
Language | : | English |
File size | : | 5502 KB |
Text-to-Speech | : | Enabled |
Enhanced typesetting | : | Enabled |
Print length | : | 221 pages |
Lending | : | Enabled |
Screen Reader | : | Supported |
Do you want to contribute by writing guest posts on this blog?
Please contact us and send us a resume of previous articles that you have written.
Book
Novel
Page
Chapter
Text
Story
Genre
Reader
Library
Paperback
E-book
Magazine
Newspaper
Paragraph
Sentence
Bookmark
Shelf
Glossary
Bibliography
Foreword
Preface
Synopsis
Annotation
Footnote
Manuscript
Scroll
Codex
Tome
Bestseller
Classics
Library card
Narrative
Biography
Autobiography
Memoir
Reference
Encyclopedia
Zetta Elliott
William Strunk Jr
Elizabeth Chandler
Yair Shapira
Jackie Oliver
Sven Talaron
Charles Bukowski
Shani Petroff
Terri Blackstock
Ann Marie Frohoff
Jemma Spark
Andrew Klavan
Bruce T Gourley
Wilbur Smith
Susan Rosson Spain
Tommalisa Lumpkin
David Ives
Webb Garrison
Nick Skelton
Leblon Carter
Light bulbAdvertise smarter! Our strategic ad space ensures maximum exposure. Reserve your spot today!
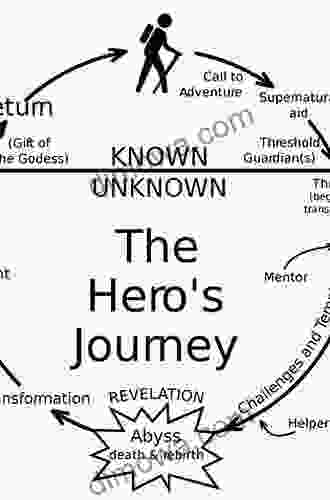

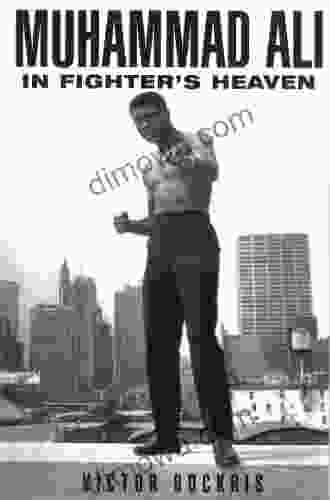

- Duncan CoxFollow ·2.6k
- Vince HayesFollow ·9.8k
- Ivan TurnerFollow ·13.6k
- Stan WardFollow ·19.2k
- Braeden HayesFollow ·16k
- Elmer PowellFollow ·6.7k
- Mark MitchellFollow ·14.6k
- Grant HayesFollow ·17.8k
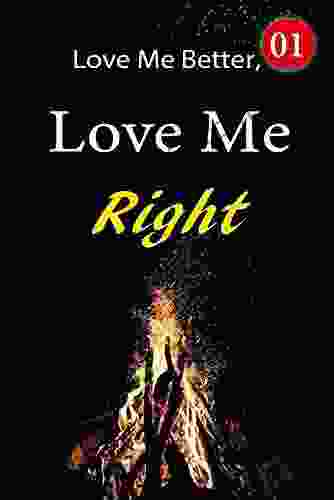

Love Me Better, Love Me Right: A Journey of...
Unveiling the Profound Power of Emotional...
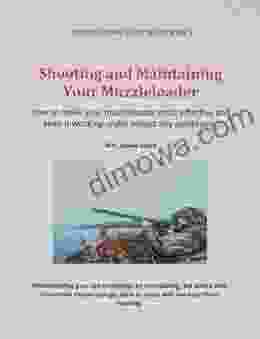

How To Make Your Muzzleloader Most Effective And Keep It...
In the realm of firearms, muzzleloaders hold...
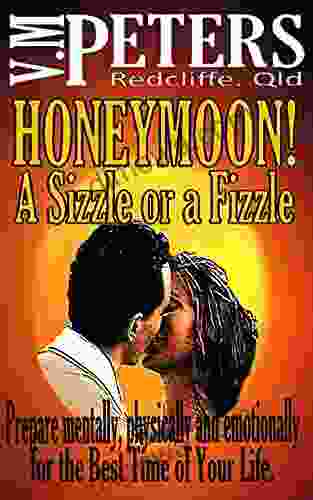

Prepare Mentally, Physically, and Emotionally for the...
Embark on a Transformative Odyssey to...
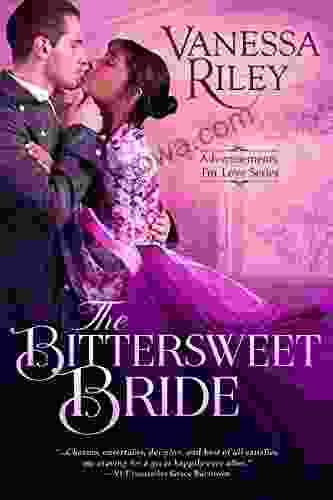

The Bittersweet Bride: Advertisements for Love
A Poignant...
4.6 out of 5
Language | : | English |
File size | : | 5502 KB |
Text-to-Speech | : | Enabled |
Enhanced typesetting | : | Enabled |
Print length | : | 221 pages |
Lending | : | Enabled |
Screen Reader | : | Supported |